The 38th edition of the State of Black America®– One Nation Underemployed: Jobs Rebuild America – underscores a reality the National Urban League knows all too well – that the major impediments to equity, empowerment and mobility are jobs, access to a living wage and wealth parity. Amidst the discourse and debate about income inequality and other economic news-of-the-day, One Nation Underemployed: Jobs Rebuild America underscores the urgency of the jobs crisis—both un-and-under-employment.
Short of an anticapitalist revolution, how do African Americans and other communities of color recover from the losses of the Great Recession and forge a path to economic stability and upward mobility? The Urban League offers great data about our crises, but comes back- for the 38th time -with a plea to and plan to work with the very same corporate and governmental forces that have created our oppressive and exploitative conditions.
SOBA2014-SinglePgs
------------------------------------
March 2014- http://www.bls.gov
Investment in higher education by race and ethnicity
Recent research studies
cover the varying perspectives on education by ethnicity and race and
the methods in which families exert influence over educational choices.
In addition, a number of studies that are rooted in Human Capital Theory
evaluate returns to schooling—education increases an individual’s
productivity and contributes to an individual’s capacity to improve his
or her financial and social well-being. Using Consumer Expenditure
Survey microdata, this article extends previous research on human
capital and investment in education by examining the patterns of
educational expenditures by race and ethnicity. The results reveal that
differences in investment arise principally from (1) differences in
college attendance and (2) the likelihood to assume educational
expenditures. Once families decide to invest in their children’s higher
education, little difference exists in the level of expenditures between
racial and ethnic groups.
“Western parents try to respect their children’s individuality, encouraging them to pursue their true passions, supporting their choices, and providing positive reinforcement and a nurturing environment. By contrast, the Chinese believe that the best way to protect their children is by preparing them for the future, letting them see what they’re capable of, and arming them with skills, work habits, and inner confidence that no one can ever take away.”
As
an example shown by the excerpt from Chua’s book, recent popular
literature discusses the varying perspectives on education by ethnicity
and race and the ways in which families exert influence over educational
choices.2
Higher education, after all, contributes to an individual’s capacity to
earn a livelihood and improve his or her financial and social
well-being, regardless of race or ethnicity.
In his book Human Capital, Nobel laureate economist Gary Becker3 articulated the premise that human capital arises out of any activity that increases individual productivity.4
Education is one such activity that increases the productivity of the
individual, requiring the direct cost of the education (tuition, books,
and housing) and the foregone earnings during education. In much the
same way that a unit of physical capital such as production machinery
generates a stream of production benefits (lightbulbs, automobiles, or
basketballs) with market value, human capital that increases as a result
of an investment in education will generate an augmented stream of
earnings and social benefits that will accrue as long as the educational
investment has market and social values.
Human
capital is primarily produced in the family and in schools. According to
Becker, parents’ altruistic investment in the child’s education depends
on their willingness to forgo their own consumption for the sake of the
child and on the likelihood that the investment will yield economic and
intrinsic personal and social benefits for the child.
In
addition, because education improves human capital, society accrues the
benefits of a more productive workforce that contributes through
specialization and innovation. Moreover, families accrue the direct and
indirect benefits of family members who are more productive and better
able to provide greater economic support to the family. Families that
invest in the human capital of their children receive the social
benefits of higher education, to include increased social opportunities
and the positive social impression made by the individual and the
family. The family is integral to the investment decision as well as the
subsequent benefits to the child, the family, and society. In the words
of Becker,5
“No discussion of human capital can omit the influence of families on the knowledge, skills, health, values, and habits of their children. Parents affect educational attainment, marital stability, propensities to smoke and to get to work on time, and many other dimensions of their children’s lives.”
At an individual
and family level, various factors influence the level of education
investment. These factors include the family socioeconomic status (such
as the amount of disposable family income and the education level of the
parents), the ability of the child to complete his or her education,
and the perceived economic and social benefits. The weight given to
investing in education is therefore related to not only the stream of
economic benefits but also the underlying characteristics of the family
that chooses to invest.
Drawing on evidence from
the Bureau of Labor Statistics’ Consumer Expenditure Survey (CE)
microdata, a household survey that provides information on the buying
habits of American consumers, this study extends previous research on
human capital and investment in education by examining differences in
educational expenditure patterns between race and ethnicity. We
disaggregate the investment decision into two separate stages and
explore any differences at each stage. First is the decision to attend
college. Second is the level of expenditures for education. An
individual who decides to attend college may incur different costs,
depending on the choice of an educational institution and family
background. Studying the amount an individual spends, given his or her
decision to attend college, may provide further insights into
differences in the educational investment decision.
In this article, parent’s education level refers to the education level of the reference parent. With the release of 2012 CE data, education of reference person was replaced by highest education level of any member in the consumer unit.
This newly defined education classification, although not used in the
analysis herein, does not change any statistical significance of the
results presented in this article.
Consequently,
this article evaluates whether race and ethnicity contribute to
differences in investments in higher education. An investment decision
includes both the decision to attend college and the amount of money
invested given that decision. Many studies stop at examining differences
in the likelihood to attend college by race and ethnicity, yet many
differences in investment may be uncovered in the level of expenditures
decided on for higher education. In this article, we first review the
discussion of returns to schooling and the decision to attend college.
Next, we describe the dataset we use for analyses. Finally, we discuss
in depth the pieces that make up the differences in an investment
decision by examining the decision to attend college by race and
ethnicity and then focusing on levels of expenditures differences for
higher education.
Returns to schooling and the decision to attend college: a literature review
Returns to schooling.
The essence of human capital theory is that investments in human
capital—schooling and training—raise a person’s income by increasing the
individual’s productivity and in satisfying society’s demand for more
highly remunerated skills. In the case of schooling, individuals forgo
money they would have earned during their working years and instead
incur direct educational costs to invest in their own human capital. The
individual’s rate of return is based on the investment value of the
gain in lifetime earnings.6
Other
research focuses on the wide variety of forms returns to education can
take, such as financial returns in the form of pay, nonmonetary
opportunities such as more job and schooling options, and nonmarket
returns; for example, the ability of the educated to use skills to
perform services for themselves such as tax preparation that would
otherwise be purchased.7
Although all of these benefits and those to society are important, the
focus of human capital returns to schooling revolves around the lifetime
earnings returns that come from additional education. A rich collection
of studies provides estimates of returns to education dating from the
late 1950s.8
These studies conclude that private rates of return vary by region,
income grouping, and gender. In addition, rates of return are highest in
primary education and diminish with additional years of education. In
the United States, the overall (primary through higher education)
private rate of return to investment in education is “on the order of 10
percent.”9
Other studies have considered race, ethnic, and gender differences in returns to schooling, in particular, higher education.10
The findings from many of these studies indicate that positive returns
to education are common across races, ethnicities, and the two genders.
Moreover, when differences in ability are considered, “little evidence
of differences in the return to school across racial and ethnic groups”
exists.11
Again, these studies, which employ different datasets at different
times, estimate annual gains from education ranging from 6 percent to 15
percent.
Taken together, a human capital
framework and the ensuing research lead to the following conclusions
about the value of schooling:
·
Additional years of schooling positively correlate with increased
earnings. This relationship holds for primary, secondary, and higher
education.
·
The decision to invest in education is one that yields a wide range of
benefits, including a positive rate of return for the individual and
society.
· The private annual rate of return varies but generally ranges from 8 percent to 10 percent.
Decision to attend college.
The likelihood of the decision to attend college hinges on the costs of
and the perceived returns (whether financial or personal) to schooling,
as just discussed. This decision is shown to influence conditions of
the family significantly, such as income, wealth, and education level.
Differences in college attendance by race and ethnicity have been well
studied, but results vary.
Studies have shown
that college attendance varies significantly by race and ethnicity.
Without controlling for family background,12
studies have consistently found that Hispanic and African American
families have lower levels of education and college attendance, while
Asians tend to have higher levels of education and college attendance.13
The focus of many other studies has been on estimating the effect of
family background factors in explaining differences in college
attendance. These studies indicate a wide range of results in explaining
college attendance gaps due to socioeconomic differences and academic
achievement. Some studies show that socioeconomic differences explain
all or at least a portion of the gap in college attendance. Other
studies have also expanded the analysis and discussed differences in
attendance between 2-year and 4-year colleges.
At
one end of the spectrum, several studies indicated that—given similar
academic achievement levels of the student and similar family
backgrounds—young African Americans were more likely to attend college.
Thomas Kane showed that at each Scholastic Assessment Test (SAT,
formerly Scholastic Aptitude Test) level, African American student
enrollment rates were higher than their White counterparts.14
Pamela Bennett and Yu Xie showed that African American students,
compared with their White counterparts, are more likely to attend
college, especially at low levels of family socioeconomic status.15
Audrey Light and Wayne Strayer showed that minorities are more likely
than their White counterparts to attend colleges of all quality levels.16
Laura Walter Perna found that African Americans are about 11 percent
more likely than Whites to enroll in a 4-year college or university in
the fall after graduating from high school.17
Many
studies indicate that college attendance differences are insignificant
after accounting for family background factors. Kane and Spizman showed
that lower average educational attainment of African Americans is the
result of differences in parental income, education, and geographical
location.18
Charles, Roscigno, and Torres showed that background inequalities
explain the entire African American–White gap in the likelihood of
college attendance.19
Sandra Black and Amir Sufi found that since the 1990s, at every point
of the socioeconomic status, African Americans were no more likely to
attend college than Whites.20 Two other studies have found that Hispanic high school graduates are as likely as Whites to attend college.21
Even
at lower levels of significance, studies generally show that
socioeconomic factors account for at least some of the difference in
college attendance among races. Min Zhan and Michael Sherraden showed
that when household assets are considered, a substantial portion of the
African American–White gap in college attendance disappears.22
Regarding
attendance at 2-year and 4-year institutions, Kao and Thompson found
that although African American and Hispanic students are more likely to
attend college than ever before, they are more likely than Whites or
Asians to attend a 2-year college than a 4-year institution.23
Cameron and Heckman found that Hispanics show the highest 2-year entry
rate compared with African Americans and Whites, which is partially
attributable to the regional concentration of Hispanics in states, such
as California and Texas, with extensive low-tuition community college
networks.24
Consumer expenditure survey dataset
The
analyses in this article are based on microdata from the Interview
Survey of the CE of the U.S. Bureau of Labor Statistics (BLS). Data from
the years 2008, 2009, and 2010 contain 90,872 observations taken from
consumers who were interviewed between January 2008 and March 2011.25
Selected subsets of the complete dataset are used for various parts of
our analyses. (See appendix A for number of observations for each
subset.) CE interviews the sample family units on the basis of a
rotating panel, surveying about 7,000 consumer units26
each quarter. Each consumer unit is interviewed once per quarter for up
to five consecutive quarters. The Interview Survey is designed to
capture expenditure data that respondents can reasonably recall for a
period of 3 months or longer. In general, data captured include
relatively large expenditures.27
This
article examines the variation across consumer units, henceforth,
“households,” at each interview, to explain differences in educational
investment among racial groups and thus the article treats each
household as a unique observation.28
All summary statistics, parameter estimates, and variances in this
article are generated using weights created through balanced repeated
replication, a procedure necessary to compute unbiased variance
estimates through the CE’s use of a stratified random sample of
geographic areas around the United States.29
The data represent the U.S. population in all analyses performed in
this article. Results are derived with a SAS macro that the BLS
developed.
To disaggregate educational
expenditures, we provide a structure that outlines the decision to incur
expenditures and describes how the CE data are organized in accordance
with this structure. (See figure 1.) First, a qualified
16–24-year-old—one who graduated from high school but does not have a
bachelor’s degree, henceforth, a “young adult,”—decides whether to go to
college. Furthermore, if he or she decides to attend college, the
educational expenditure may be made by the parent(s), young adult, both,
or neither. For completeness, we discuss three types of households
relevant to educational expenditures:
· Type 1—parent household without young adults residing in the household
· Type 2—young adult household
· Type 3—parent household with young adult(s) residing in the household
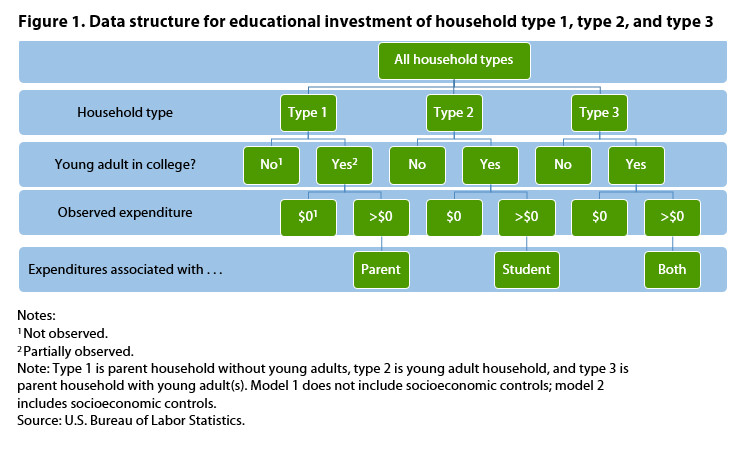
Households
are identified as type 1 (78,686 observations) if no young adult member
is acknowledged, during the interview, to reside in that household.
Type 1 households include families (1) that do not have any young adult
members (regardless of residence) and (2) those who have one (or more)
young adult member(s) but who may be away (for college or living
separately), although we cannot distinguish between these two subtypes
in the data. Households identified as type 2 (4,099 observations)
comprise young adult members who are the head of their household. This
type includes those who are living apart30
from parents such as a college student. Type 3 (8,087 observations)
households are identified as those with at least one young adult member
who is not the head of a separate household and has at least one other
older member (e.g., parent).
For type 1
households, we do not observe whether a young adult is away at college;
we only know that, when we observe a positive expenditure for an
unobserved individual who is away from home, an individual (likely to be
a young adult) is away at college.31 For type 2 households, we observe the young adult’s college attendance decision32
and his or her own out-of-pocket expenditure for higher education. We
do not, however, observe the young adult’s family background. For type 3
households, we observe both the young adult’s college attendance
decision and the household expenditure for higher education.
Race and ethnicity.
An individual’s race is identified as White, African American, Asian,
or “other.” “Other” includes Native American, Pacific Islander, and
multiracial. Ethnicity is classified as Hispanic and non-Hispanic. For
the purpose of this article and because of limitations of sample size,
Hispanic origin will be considered as a subset of only the White racial
group. African American Hispanics and Asian Hispanics are grouped into
“other.” Thus, the racial and ethnic groups used for this article are
White (White non-Hispanics), Hispanics (White Hispanics), African
Americans (African American non-Hispanics), Asians (Asian
non-Hispanics), and other.33
Data control variables.
To control for variations in the data that may inadvertently bias model
results, we include indicators for year of interview, month of
interview, month of expenditure, part-time student, and female.
Socioeconomic variables.
For type 1 and type 3 households, factors associated with family
background are observed, including education of the parent(s) and total
outlays34 of the household, which is used as a proxy for permanent income.35 (See appendix B for more details.)
Other variables and model selection.
Additional variables such as occupation and assets were available to be
used in various parts of the analyses; however, the selection of
covariates to use in each model was based on a combination of intuition
and empiricism. Variables that had an economic relation and linearly
related to the dependent variable were added to each model. Selection
criteria36 were then applied and resulted in the final models that follows.
College attendance rates
Prior
to deciding on an amount to spend for higher education, individuals and
families must first decide whether a member of the family is to attend
college. As just discussed, the decision to attend is influenced by many
factors, such as family income, race, their parents’ education, and
wealth.
In our dataset, more than half of qualified young adults37
were in college, of which 83 percent were enrolled full time. Our data
show that Hispanics (45 percent) and African Americans (43 percent)
generally have lower college attendance rates than Whites (55 percent)
or Asians (73 percent). As with previous studies, family background,
such as their parents’ education and income, was significantly
associated with the likelihood of attending college. As parent’s
education level and income increase, the likelihood of a young adult
attending college significantly increases. The extent to which
socioeconomic differences explain differences in college attendance
among racial and ethnic groups varies among models, but socioeconomic
differences were generally found to account for some of the observed
differences.38
(See appendix C, table C-1.) Part-time college attendance rates for
Whites, African Americans, and Asians were between 7 percent and 8
percent, while Hispanics had a 14 percent part-time attendance rate.
(See figures 2 and 3.)
Tuition expenditure patterns for attendees
At the aggregate level, as with published CE tables,39
Hispanic and African American households have a lower average household
expenditure than White households do, whereas Asian households have a
higher average household expenditure. (See table 1.) However, these
overall averages are misleading, because they do not distinguish those
students who are attending college from those who are not.
Race or ethnicity | Mean | Standard error |
---|---|---|
White | 409.2 | 27.4 |
Hispanic | 177.3 | 49.2 |
African American | 125.3 | 18.5 |
Asian | 644.3 | 112.1 |
Note: “Other” group is omitted.
Source: U.S. Bureau of Labor Statistics. |
Once
an individual has decided to attend college, he or she must decide on
the amount to invest in higher education. In this section, we first
discuss the characteristics of households with college-attending
students. We then model the level of out-of-pocket tuition expenditures40
for those with a positive expenditure. Finally, we examine factors that
are associated with a zero or unobserved out-of-pocket tuition
expenditures.41
Profile of households with college-attending students.
Among households with college-attending students, family
characteristics vary significantly among racial and ethnic groups. Table
2 shows the characteristics of type 3 households—those with one or more
parents and one or more students—by race and ethnicity in the dataset
used for the analyses.
Education.
Compared with Hispanic and African American parents, White and Asian
parents of college-attending students have higher levels of education.
Whereas 33 percent of White parents and 30 percent of Asian parents
obtained a bachelor’s degree or higher, 18 percent of African American
parents and 10 percent of Hispanic parents obtained the same degree. At
the lower end of the education scale, 5 percent of White parents and 8
percent of Asian parents did not receive a high school diploma. In
contrast, 12 percent of African American and 32 percent of Hispanic
parents did not receive a high school diploma.
Education of parents | White | Hispanic | African American | Asian |
---|---|---|---|---|
Less than high school (percent) | 5 | 32 | 12 | 8 |
High school (percent) | 27 | 24 | 27 | 32 |
Some college, associate’s degree (percent) | 36 | 34 | 42 | 30 |
Bachelor’s degree (percent) | 23 | 7 | 13 | 17 |
Graduate degree (percent) | 10 | 3 | 5 | 13 |
Annual income (annualized total outlays) (dollars)(1) | 67,269 | 48,327 | 48,868 | 58,667 |
Have any tuition expenditures (percent) | 28 | 18 | 14 | 28 |
Average quarterly tuition (dollars) | 2,806 | 1,356 | 1,606 | 3,397 |
Female student (percent) | 58 | 62 | 58 | 47 |
Notes: (1) Consumer unit type 3 households only (parents with young adults). Note: The term “total outlays” is a proxy for permanent income; we obtained annualized outlays by multiplying quarterly outlays by 4. Source: U.S. Bureau of Labor Statistics. |
Income. Asian parents of college-attending students reported 13 percent less permanent income42
($58,700) than White parents ($67,300). Relative to the income of White
parents, Hispanic and African American parents had 28 percent and 27
percent less annual income, or $48,300 and $48,900, respectively.
Positive tuition expenditure.
Asian households were as likely as White households to have a positive
expenditure for education. However, Hispanics households were more than
one-third less likely than White households to have a positive
expenditure, while African Americans were only about half as likely.
Average tuition expenditure.
Of the households with positive tuition expenditures, Asians reported
21 percent higher expenditures than Whites. Hispanics and African
Americans reported 52 percent and 43 percent, respectively, lower
expenditures than Whites reported.
While
Hispanics and African Americans households with a student in college
had, on average, a lower probability of having a positive expenditure
and a lower amount of expenditure, they also had lower education and
lower total outlays. To ascertain whether certain racial or ethnic
groups tended to spend less (or more) on education because of their
average socioeconomic differences, we performed a regression analysis.
Observed out-of-pocket tuition expenditures.
We constructed a model for out-of-pocket positive tuition expenditures
and used the method of ordinary least squares to estimate not only the
relationship between race and ethnicity, but also socioeconomic
characteristics, and the level of positive tuition expenditures. (See
box that follows.)
A model for tuition expenditures
Because expenditures are truncated at zero, are clustered around lower values, and have a long tail (are right-skewed), a transformation of the dependent variable is applied to approximate normality. For each model, an optimal Box-Cox transformation is applied to distribute tuition expenditures more normally and to stabilize variance.1We start with an ordinary least squares model of (transformed) positive expenditures regressed on race and ethnicity, with data controls in which

where Yλ is a vector of (transformed) observed tuition expenditure for higher education; X is a matrix of indicators for race and ethnicity; β is a vector of corresponding parameter estimates; Z is a matrix of data controls, including indicators for interview year, interview month, tuition expenditure month, female, and part-time student; γ is a vector of corresponding parameter estimates; and ε is a vector of errors.
We then add socioeconomic factors to equation (1), yielding

where W is a vector of family background characteristics, including parent’s education level and annual permanent income,2 and η is a vector of corresponding parameter estimates.
Notes:
1 The optimal λ for consumer unit types 1, 2, and 3 are 0.12, 0.24, and 0.08, respectively.
2 The logarithm of income (annualized outlays) is used to overcome nonlinearity between permanent income and tuition expenditures.
The
regression model results indicate significant differences in tuition
expenditures by income, education, and race and ethnicity.
Income.
Family income has a significant effect on educational expenditures.
Ceteris paribus, for every $10,000 increase in annual permanent income,
average annual tuition expenditures increase between $200 and $400 for
type 1 households (parents without young adults) and between $120 and
$360 for type 3 households (parents with young adults).43 (See appendix C, table C-2.)
Education.
At household education levels of bachelor’s degree and below,
differences in tuition expenditures are insignificant. On average,
households with parents having a graduate degree had college tuition
expenditures 40 percent to 80 percent higher than households with
parents not having a graduate degree.44
Race and ethnicity.
Differences in the level of expenditure for tuition are most pronounced
for student-only households (type 2). Hispanic students, on average,
spent half the amount that an average White student spent on tuition. On
the other hand, Asians spent nearly twice as much as their White
counterpart. However, the extent to which family characteristics explain
the differences in expenditures remains uncertain for type 2
(student-only) households because these characteristics are unobserved
in the data. Also, because of the small sample size of African American
type 2 households, expenditure differences between this group and Whites
are not statistically significant.
For
parent-only (type 1) households, no significant differences in
expenditures were found between racial and ethnic groups. For student
and parent joint (type 3) households, Hispanics had 38 percent lower
tuition expenditures than Whites. However, when controlling for the
socioeconomic differences between Hispanics and Whites, we found that
Hispanics’ lower expenditures were not statistically significant.45
This finding indicates that permanent income and education level
effects accounted for nearly all the observed differences in tuition
expenditures between Hispanics and Whites. (See table 3 and appendix C,
table C-3.)
Variable | Type 1 (model 1) | Type 1 (model 2) | Type 2 (model 1) | Type 3 (model 1) | Type 3 (model 2) |
---|---|---|---|---|---|
Race or ethnicity | |||||
Hispanic
| –0.088 | –0.091 | –0.902 | –0.066 | –0.037 |
(0.089) | (0.069) | (0.354)(1) | (0.020)(2) | (0.022)(3) | |
African American
| 0.002 | 0.055 | –0.640 | –0.036 | –0.020 |
(0.076) | (0.064) | (0.528) | (0.024) | (0.025) | |
Asian
| 0.001 | 0.007 | 0.952 | 0.043 | 0.056 |
(0.057) | (0.062) | (0.407)(1) | (0.028) | (0.033) | |
Data controls | Yes | Yes | Yes | Yes | Yes |
Socioeconomic controls | No | Yes(4) | No(5) | No | Yes |
Notes: (1) Significant at the 5-percent α level. (2) Significant at the 1-percent α level. (3) Significant at the 10-percent α level. (4) In-college part time and female not available. See appendix C, table C-3. (5) Socioeconomic controls not available. Note: Standard errors are in parenthesis. Type 1 is parent household without (visible) young adults, type 2 is young adult household, and type 3 is parent household with young adult(s). Model 1 does not include socioeconomic controls; model 2 includes socioeconomic controls. Source: U.S. Bureau of Labor Statistics. |
Unobserved and zero out-of-pocket expenditures.
The previous section addressed positive tuition expenditure
differences, yet another important aspect to address is the likelihood
of having a positive (and unobserved) expenditure.46A
household with a young adult attending college may not have an observed
positive expenditure for tuition. Many factors may prevent an
expenditure from being observed. Examples include scholarships or some
other financial aid covering all tuition fees,47
someone outside of the household covering the expenditure (especially
for type 2 households), or someone covering a tuition expenditure in a
month outside of the interview coverage months. Although the reasons for
zero out-of-pocket expenditures are unknown in the data, we examine
factors that are associated with the likelihood of having a positive
tuition expenditure for types 2 and 3, the households in which a young
adult in college is present. This last piece of modeling zero
out-of-pocket expenditures is necessary and important to complete the
analysis of investment in higher education. The box that follows
describes our model for zero out-of-pocket tuition expenditures.
A model for zero expenditures
The response variable, regardless of whether a tuition expenditure is positive or zero, is a binary response. We use a logit1 model with data controls for type 2 and type 3 households as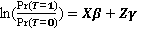
where T indicates positive tuition expenditure; X is a matrix of indicators for race and ethnicity; β is a vector of corresponding parameter estimates; Z is a matrix of data controls, including indicators for interview year, interview month, female, and part-time student; and γ is a vector of corresponding parameter estimates. The response in a logistic regression is modeled as log-odds, that is, the log of the ratio of the probability of a positive expenditure versus a zero expenditure.
For type 3 households, we then included socioeconomic controls as

where in this equation, X, β, Z, and γ are as in equation (1); W is a matrix of family background characteristics, including parent’s education level and logarithm of total quarterly outlays, which are used as a proxy for permanent income; and η is a vector of corresponding parameter estimates.
Note:
1 We also used probit and linear probability models. The results were similar.
As
with the results for the levels of expenditures, results obtained from
models of zero expenditure indicate that income, education, and race and
ethnicity are important determinates of whether a household will have
positive expenditures.
Income.
Not surprisingly, permanent income is significantly associated with the
likelihood of having any tuition expenditure. The probability of having
out-of-pocket tuition expenditure increases with family income level.
This relationship is expected because families with lower permanent
income tend to receive more financial aid,48
increasing the likelihood of complete tuition coverage. Compared with
families with an annual permanent income of $20,000 or less, families
with $120,000 or more with a college-going child are about 6.5 times
more likely to have tuition expenditure. (See figure 4.) Even after
controlling for other covariates, such as race, other socioeconomic
factors, and data controls, the income effect remains highly
significant. (See appendix C, table C-2.)
Education.
As expected, as parents’ education level increases, the probability of
having positive tuition expenditure increases. (See figure 5.) Even when
other factors such as permanent income are controlled, the probability
still increases with education level.49 (See appendix C, table C-2, for results obtained from the model.)
Race and ethnicity.
Even when differences in family background are controlled, our model
yields results which indicate that, of those households with one or more
members who attend college, African American households are
significantly less likely to have an observed positive expenditure for
tuition compared with White households. Asian households show a
marginally significantly higher probability of having a positive
expenditure.50 (See table 4 and appendix C, table C-2.)
Variable | Type 2 (model 3): without socioeconomic controls | Type 3 (model3): without socioeconomic controls | Type 3 (model 4): with socioeconomic controls |
---|---|---|---|
Race or ethnicity | |||
Hispanic
| 0.134 | –0.194 | –0.029 |
(0.203) | (0.160) | (0.151) | |
African American
| –1.154 | –0.560 | –0.476 |
(0.309)(1) | (0.176)(2) | (0.163)(2) | |
Asian
| 0.648 | 0.279 | 0.233 |
(0.336)(3) | (0.141)(4) | (0.124)(3) | |
Data controls | Yes | Yes | Yes |
Socioeconomic controls | No(5) | No | Yes |
Notes: (1) Significant at the 0.1-percent α level. (2) Significant at the 1-percent α level. (3) Significant at the 10-percent α level. (4) Significant at the 5-percent α level. (5) Socioeconomic controls not available. Note: Standard errors are in parenthesis. Type 2 is young adult household, and type 3 is parent household with young adult(s). Source: U.S. Bureau of Labor Statistics. |
DIFFERENCES
IN INVESTMENT IN HIGHER EDUCATION between racial and ethnic groups
arise from differences in college attendance, the level of expenditures
for higher education and, to a greater extent, the likelihood of having
positive education expenditures. Some of the differences, as shown in
table 5, can be attributed to differences in family socioeconomic
status, such as parent’s education level and permanent income. In
narrative terms, our results are as follows:
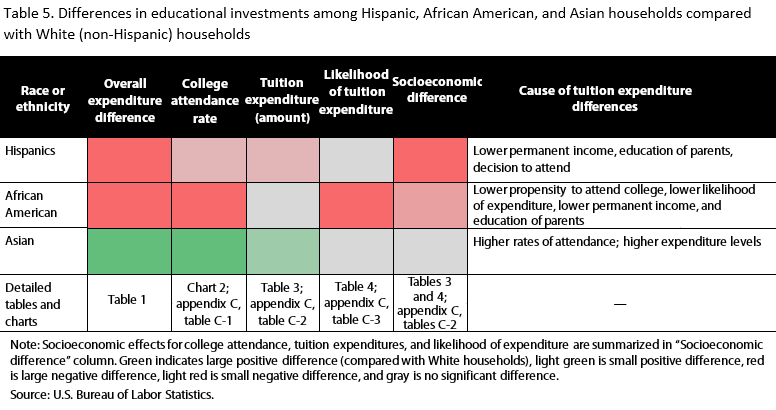
·
Relative to White households, Hispanic households tend to have lower
rates of college attendance and some evidence shows lower levels of
tuition expenditures, as well. Our results indicate that the overall
lower levels of household tuition expenditures for Hispanics are due
primarily to generally lower levels of permanent income, parent’s
education level, the decision to attend college and, to some extent, the
levels of tuition expenditure of students. However, after accounting
for differences in education and permanent income, Hispanic households
do not differ significantly either in the likelihood of having any
tuition expenditures or in the level of expenditures.
·
African American young adults tend to have lower rates of college
attendance and, among those who decide to attend college, a lower
probability of having any tuition expenditure, even when family
permanent income and education level are considered. However, of those
who have any tuition expenses, the levels of expenditures are not
significantly different from those of White families. The primary
contributing factors to lower levels of household tuition expenditure by
African Americans are socioeconomic differences, a lower likelihood to
attend college, and the higher likelihood of having no expenditures even
when one decides to attend college.
·
Asian young adults have higher rates of college attendance than any
other race or ethnicity. Asian parents do not have a significantly
higher level of tuition expenditures. However, some evidence points to
higher levels of expenditures from students in student-only households.
These results suggest that Asian households’ overall higher expenditures
for education are primarily due to the higher rates of college
attendance in Asian families, and, to some extent, the higher levels of
tuition expenditures by Asian student-only households.
With
a variety of factors contributing to the overall decision of college
investment, differences arise among racial and ethnic groups.
Notwithstanding the complexity of socioeconomic factors, our findings
suggest that differences in permanent income and education level of
parents are important in the decision to attend college, in the level of
expenditures, and in the likelihood of having a positive expenditure
for education. Controlling for these important factors, we find that
whereas the decision whether to invest may be different for Asians,
African Americans, and Hispanics, the amount of investment, for the most
part, is not significantly different from that of Whites.
Previous
studies have shown that similar returns to schooling exist across
different races and ethnicities, even if the likelihood to attend
college is different. However, given the decision to invest and given
evidence of positive expenditures, we found no significant differences
in the level of investment. These results suggest that, although the
perceived returns to schooling may be different for different races and
ethnicities, the investment value of education for those who choose to
invest is unambiguous, both in perception and in effect. Returning to
the earlier discussion of higher education and human capital, we
conclude that once a decision to invest in higher education is made and
positive expenditures are registered by a family, little difference
exists in the amount for education investments between the racial and
ethnic groups studied. In other words, we find that all racial and
ethnic groups believe in and invest in the human capital idea that
investments in higher education are economically beneficial to their
children.
Acknowledgments
The authors
would like to thank BLS Senior Economist Geoffrey Paulin from the Office
of Prices and Living Conditions for his invaluable comments, review,
guidance, and patience through many revisions of this article; BLS
Branch Chief and Supervisory Economist Steve Henderson, BLS Supervisory
Economist Bill Passero, and BLS Economists Adam Reichenberger and
Lucilla Tan, all also from the Office of Prices and Living Conditions;
BLS Supervisory Economist Martin Kohli from the New York Regional Office
for Economic Analysis and Information, BLS Deputy Associate
Commissioner Michael Strople from the Office of Field Operations, BLS
Supervisory Economist Amar Mann from the San Francisco Regional Office,
BLS Associate Commissioner Jay Mousa from the Office of Field
Operations, and Research Economist Harley Frazis from the Office of
Employment and Unemployment Statistics, for their review and comments;
and Regina Wu, formerly an intern at BLS, for her research assistance,
comments, and review.
Appendix A: Number of observations
Race or ethnicity | All observations by type | In college by type | Observed positive tuition expenditure by type | |||||
---|---|---|---|---|---|---|---|---|
1 | 2 | 3 | 2 | 3 | 1 | 2 | 3 | |
White | 55,301 | 2,956 | 4,539 | 2,070 | 2,192 | 868 | 476 | 644 |
Hispanic | 8,951 | 404 | 1,707 | 165 | 783 | 41 | 28 | 130 |
African American | 9,126 | 454 | 1,130 | 205 | 485 | 47 | 13 | 66 |
Asian | 3,608 | 169 | 461 | 144 | 314 | 57 | 41 | 88 |
Other | 1,700 | 116 | 250 | 71 | 118 | 12 | 10 | 29 |
Total | 78,686 | 4,099 | 8,087 | 2,655 | 3,892 | 1,025 | 568 | 957 |
Note:
Type 1 is parent household without young adults, type 2 is young adult
household, and type 3 is parent household with young adult(s). Source: U.S. Bureau of Labor Statistics. |
Appendix B: Variables
Variable | Values and range | Notes |
---|---|---|
Consumer unit type | 1: No qualified 16–24-year-old in consumer unit | Consumer unit type |
2: Qualified 16–24-year-old without parent(s) in consumer unit | ||
3: Qualified 16–24-year-old with parent(s) in consumer unit | ||
In college | 1: In college full time | For qualified 16–24-year-olds only |
2: In college part time | ||
3: Not in college | ||
Tuition expenditure for higher education | [$0, ∞) ϵ ℝ | Quarterly expenditure |
Tuition indicator | 1: Tuition > 0 | — |
0: Tuition = 0 or blank | ||
Tuition expenditure month | [1, 13] ϵ ℕ | Month in which the expenditure was made; month 13 indicates same amount each month |
Race and ethnicity | 1: White non-Hispanic | Race and ethnicity of head of household; other includes Native American, Pacific Islander, and multiracial |
2: White Hispanic | ||
3: African American non-Hispanic | ||
4: Asian non-Hispanic | ||
5: Other | ||
Female
| 0: Male | Gender of qualified 16–24-year-olds |
1: Female | ||
Year | [2008, 2011] ϵ ℕ | Year of interview |
Interview month | [1, 12] ϵ ℕ | Month in which the interview was conducted |
Education level
| 1: No high school graduate | For types 1 and 3 consumer unit head of households only |
2: High school graduate | ||
3: Some college, associate’s degree | ||
4: Bachelor’s degree | ||
5: Master’s, professional, or doctorate degree | ||
Total outlays less tuition expenditures for higher education | [$0, ∞) ϵ ℝ | Quarterly outlays; for types 1 and 3 consumer unit head of households only; proxy for permanent income |
Source: U.S. Bureau of Labor Statistics. |
Appendix C: Additional tables
Variable | Type 3: without socioeconomic covariates | Type 3: with socioeconomic covariates | ||
---|---|---|---|---|
Estimate | Standard error | Estimate | Standard error | |
Intercept | 0.047 | (0.065) | –3.027 | (0.840)(1) |
Hispanic | –.206 | (.131) | –.037 | (.138) |
African American | –.315 | (.113)(2) | –.249 | (.114)(3) |
Asian | .737 | (.149)(1) | .634 | (.157)(1) |
Other | –.089 | (.246) | –.077 | (.267) |
Interview year
| ||||
2008
| –.228 | (.064)(1) | –.229 | (.066)(1) |
2009
| –.062 | (.052) | –.061 | (.055) |
2000
| .134 | (.044)(2) | .151 | (.046)(1) |
Interview month
| ||||
January
| –.015 | (.061) | –.027 | (.061) |
February
| –.059 | (.057) | –.051 | (.061) |
March
| –.056 | (.065) | –.061 | (.066) |
April
| –.034 | (.066) | –.032 | (.072) |
May
| .028 | (.082) | .040 | (.083) |
June
| .059 | (.074) | .060 | (.074) |
July
| –.122 | (.064)(4) | –.136 | (.067)(3) |
August
| .019 | (.061) | .042 | (.065) |
September
| .040 | (.078) | .005 | (.074) |
October
| .013 | (.066) | .014 | (.074) |
November
| .024 | (.068) | .042 | (.070) |
Education
| ||||
Less than high school
| — | — | –.538 | (.084)(1) |
High school
| — | — | –.279 | (.078)(1) |
Some college, associate’s degree
| — | — | .064 | (.066) |
Graduate degree
| — | — | .472 | (.142)(1) |
Log income | — | — | .340 | (.091)(1) |
Notes: (1) Significant at the 0.1-percent α level. (2) Significant at the 1-percent α level. (3) Significant at the 5-percent α level. (4) Significant at the 10-percent α level. Note: Type 3 is parent household with young adult(s). Source: U.S. Bureau of Labor Statistics. |
Variable | Type 1 (model 1) | Type 1 (model 2) | Type 2 (model 1) | Type 3 (model 1) | Type 3 (model 2) | |||||
---|---|---|---|---|---|---|---|---|---|---|
Estimate | Standard error | Estimate | Standard error | Estimate | Standard error | Estimate | Standard error | Estimate | Standard error | |
Intercept | 2.239 | (0.105)(1) | 0.383 | (0.293) | 5.543 | (0.718)(1) | 1.777 | (0.051)(1) | 1.310 | (0.145)(1) |
Hispanic | –.088 | (.089) | –.091 | (.069) | –.902 | (.354)(2) | –.066 | (.020)(3) | –.037 | (.022)(4) |
African American | .002 | (.076) | .055 | (.064) | .640 | (.528) | –.036 | (.024) | –.020 | (.025) |
Asian | .001 | (.057) | .007 | (.062) | .952 | (.407)(2) | .043 | (.028) | .056 | (.033) |
Other | .045 | (.188) | .081 | (.142) | .717 | (.510) | –.095 | (.042)(2) | –.073 | (.041)(4) |
Interview year
| ||||||||||
2009
| .035 | (.059) | .041 | (.059) | –.326 | (.349) | –.020 | (.028) | –.010 | (.023) |
2010
| .033 | (.061) | .015 | (.064) | –.114 | (.382) | –.016 | (.033) | –.003 | (.029) |
2011
| .026 | (.059) | .020 | (.056) | –.230 | (.505) | –.007 | (.027) | .006 | (.022) |
Interview month
| ||||||||||
January
| .054 | (.092) | .031 | (.086) | .368 | (.809) | –.020 | (.051) | –.015 | (.053) |
February
| .155 | (.089)(4) | .119 | (.078) | –.784 | (.621) | –.001 | (.039) | .002 | (.039) |
March
| .020 | (.082) | –.007 | (.075) | –.991 | (.659) | –.027 | (.039) | –.024 | (.038) |
April
| .019 | (.099) | .026 | (.086) | –.751 | (.638) | –.015 | (.038) | –.005 | (.037) |
May
| .041 | (.110) | .027 | (.104) | –.992 | (.651) | –.018 | (.049) | –.011 | (.050) |
June
| –.107 | (.092) | –.096 | (.085) | –.091 | (.580) | –.060 | (.040) | –.047 | (.038) |
July
| –.031 | (.095) | –.024 | (.090) | –.050 | (.608) | –.122 | (.055)(2) | –.125 | (.052)(2) |
August
| –.009 | (.096) | –.017 | (.086) | .082 | (.749) | –.048 | (.050) | –.044 | (.049) |
September
| .021 | (.069) | .025 | (.064) | –.186 | (.580) | –.051 | (.033) | –.047 | (.029) |
October
| .005 | (.077) | .016 | (.066) | .082 | (.522) | –.044 | (.035) | –.041 | (.033) |
November
| .020 | (.064) | .027 | (.058) | –.028 | (.547) | –.035 | (.030) | –.035 | (.027) |
Expenditure month
| ||||||||||
January
| .267 | (.050)(1) | .233 | (.049)(1) | 1.647 | (.375)(1) | .069 | (.025)(3) | .073 | (.023)(3) |
February
| .055 | (.065) | .034 | (.058) | .090 | (.586) | .034 | (.023) | .035 | (.021) |
March
| .205 | (.060)(3) | .200 | (.057)(3) | .490 | (.773) | .037 | (.030) | .030 | (.030) |
April
| .224 | (.066)(3) | .189 | (.061)(3) | .171 | (.444) | .057 | (.035) | .051 | (.035) |
May
| .113 | (.068) | .088 | (.062) | –.650 | (.573) | .041 | (.030) | .036 | (.029) |
June
| .084 | (.061) | .046 | (.052) | –.245 | (.310) | .099 | (.032)(3) | .104 | (.030)(3) |
July
| .279 | (.056)(1) | .257 | (.046)(1) | .369 | (.669) | .094 | (.042)(2) | .077 | (.040)(4) |
August
| .294 | (.048)(1) | .231 | (.044)(1) | 1.129 | (.601)(4) | .097 | (.026)(1) | .095 | (.027)(1) |
September
| .210 | (.051)(1) | .171 | (.043)(1) | .418 | (.402) | .073 | (.029)(2) | .074 | (.029)(2) |
October
| .141 | (.053)(2) | .133 | (.043)(3) | .282 | (.484) | .016 | (.032) | .023 | (.031) |
November
| –.017 | (.061) | –.025 | (.057) | .604 | (.588) | –.039 | (.041) | –.033 | (.040) |
December
| .273 | (.047)(1) | .265 | (.043)(1) | .481 | (.419) | .072 | (.015)(1) | .065 | (.014)(1) |
In college part time | — | — | — | — | –.912 | (.282)(3) | –.080 | (.017)(1) | –.067 | (.019)(3) |
Female | — | — | — | — | .028 | (.156) | –.014 | (.013) | –.011 | (.013) |
Education
| ||||||||||
Less than high school
| — | — | –.014 | (.070) | — | — | — | — | –.007 | (.032) |
High school
| — | — | –.024 | (.044) | — | — | — | — | –.003 | (.016) |
Some college, associate’s degree
| — | — | .095 | (.028)(3) | — | — | — | — | .062 | (.018)(3) |
Graduate degree
| — | — | .161 | (.046)(3) | — | — | — | — | .076 | (.022)(3) |
Log income | — | — | .183 | (.028)(1) | — | — | — | — | .043 | (.016)(3) |
Notes: (1) Significant at the 0.1-percent α level. (2) Significant at the 5-percent α level. (3) Significant at the 1-percent α level. (4) Significant at the 10-percent α level. Note: Type 1 is parent household without young adults, type 2 is young adult household, and type 3 is parent household with young adult(s). Model 1 does not include socioeconomic controls; model 2 includes socioeconomic controls. Source: U.S. Bureau of Labor Statistics. |
Variable | Type 2 (model 3) | Type 3 (model 3) | Type 3 (model 4) | |||
---|---|---|---|---|---|---|
Estimate | Standard error | Estimate | Standard error | Estimate | Standard error | |
Intercept | –1.906 | (0.254)(1) | –1.257 | (0.101)(1) | –8.325 | (0.971)(1) |
Hispanic | .134 | (.203) | –.194 | (.160) | –.029 | (.151) |
African American | –1.154 | (.309)(1) | –.560 | (.176)(2) | –.476 | (.163)(2) |
Asian | .648 | (.336)(3) | .279 | (.141)(4) | .233 | (.124)(3) |
Other | –.050 | (.453) | .082 | (.183) | .044 | (.195) |
In college part time | –.042 | (.179) | –.474 | (.145)(2) | –.358 | (.153)(4) |
Female | .033 | (.162) | –.094 | (.090) | –.066 | (.096) |
Interview year
| ||||||
2008
| .205 | (.131) | .072 | (.091) | .079 | (.092) |
2009
| .091 | (.095) | .158 | (.071)(4) | .181 | (.072)(4) |
2000
| –.023 | (.135) | –.117 | (.055)(4) | –.094 | (.057)(3) |
Interview month
| ||||||
January
| –.477 | (.317) | –.250 | (.156) | –.295(1) | (.156)(3) |
February
| .709 | (.221)(2) | .361 | (.145)(4) | .461 | (.155)(2) |
March
| .452 | (.151)(2) | .465 | (.120)(1) | .471 | (.126)(1) |
April
| .337 | (.209) | .287 | (.142)(4) | .293 | (.149)(4) |
May
| –1.011 | (.315)(2) | –.937 | (.147)(1) | –.912 | (.151)(1) |
June
| –.372 | (.394) | –.481 | (.161)(2) | –.505 | (.170)(2) |
July
| .268 | (.245) | –.183 | (.099)(3) | –.248 | (.104)(4) |
August
| –.479 | (.534) | –.599 | (.170)(1) | –.584 | (.166)(1) |
September
| .283 | (.146)(3) | .453 | (.129)(1) | .413 | (.134)(2) |
October
| .550 | (.190)(2) | .568 | (.123)(1) | .528 | (.130)(1) |
November
| .405 | (.154)(2) | .541 | (.138)(1) | .611 | (.138)(1) |
Education
| ||||||
Less than high school
| — | — | — | — | –.382 | (.176)(4) |
High School
| — | — | — | — | –.236 | (.096)(4) |
Some college, associate’s degree
| — | — | — | — | .031 | (.087) |
Graduate degree
| — | — | — | — | .469 | (.178)(2) |
Log income | — | — | — | — | .745 | (.100)(1) |
Notes: (1) Significant at the 0.1-percent α level. (2) Significant at the 1-percent α level. (3) Significant at the 10-percent α level. (4) Significant at the 5-percent α level. Note: Type 2 is young adult household and type 3 is parent household with young adult(s). Model 3 does not include socioeconomic controls; model 4 includes socioeconomic controls. Source: U.S. Bureau of Labor Statistics. |
Notes
1 New York: Penguin Press, 2011.
2 See also Sandra Tsing Loh, “My Chinese American problem—and ours,” The Atlantic Monthly, April 2011, p. 83–91.
3Gary S. Becker, Human Capital: a theoretical and empirical analysis, with special reference to education, 3rd ed. (Chicago, IL, University of Chicago Press, 1994).
4 Jacob Mincer’s book Schooling, experience, and earnings (New York: Columbia
University Press, 1974) is also credited with providing a theoretical
and empirical basis for evaluating human capital and earnings.
5Gary S. Becker, “Human capital,” in David R. Henderson, The concise encyclopedia of economics, Library of Economics and Liberty (Indianapolis: Liberty Fund, 2008), http://www.econlib.org/library/Enc/HumanCapital.html.
6Societies
invest in human capital in the form of educational facilities,
programs, and subsidies. The social rate of return is based on the
benefits to society of having a more highly educated workforce, less the
cost of providing the educational services required to achieve those
benefits. In evaluating the rate of return to schooling, human capital
theory factors in the effects of cognitive ability and technological
change on individual human capital and economic growth. Briefly, the
reasoning is that the cognitive ability of the individual contributes to
the returns to schooling because those with higher cognitive abilities
select schooling over paid work, and therefore, the observed returns
actually relate to the individual’s innate abilities rather than the
level of schooling. The difficulty in distinguishing the contribution of
ability from that of schooling has led some economists to conclude that
“education and cognitive ability are so strongly associated that the
wage effects of the two cannot be separated for all groups . . . . The
real problem is that ability and schooling appear to be inseparable—all
interaction and not main effects—even if ability is perfectly observed.”
See James Heckman and Edward Vytlacil, “Identifying the role of
cognitive ability in explaining the level of and change in the return to
schooling,” Working Paper 7820 (Cambridge, MA: National Bureau of
Economic Research, August 2000), p. 18. Other researchers have also
evaluated the effects of “signaling” ability on rates of return to
schooling. The idea is that, in the absence of another tangible measure
of ability, achievements in higher education allow employers to sort
prospective employees on the basis of the presumed “signal” that the
individual has enhanced productivity. A National Bureau of Economic
Research study concluded that although high school graduates returns to
ability are negligible, college graduation “plays more than just a
signaling role in the determination of wages. . . . Graduation from
colleges allows individuals to directly reveal their ability to
potential employers.” In other words, differences in ability do not lead
to significant wage differences for high school graduates without
experience but are more tangible among college graduates and are
reflected in differences in wages. Moreover, among college graduates, no
estimated differences exist in wages or returns to ability by race. For
more information, see Peter Arcidiacono, Patrick Bayer, and Aurel
Hizmo, “Beyond signaling and human capital: education and revelation of
ability,” Working Paper 13951 (Cambridge, MA: National Bureau of
Economic Research, April 2008).
7Burton A. Weisbrod, “Education and investment in human capital,” part 2, “Investment in human beings,” Journal of Political Economy, October 1962, pp. 106–123.
8 For
a useful survey of studies in various countries over nearly 50 years,
see George Psacharopoulos and Harry Anthony Patrinos, “Returns to
investment in education: a further update,” Education Economics 12, no. 2 (September 2002), pp. 111–134.
9 Ibid., p. 116.
10 Fred
Hines, Luther Tweeten, and Martin Redfern, “Social and private rates of
return to investment in schooling, by race-sex groups and regions,” Journal of Human Resources, Summer 1970, pp. 318–340; Susan Averett and Sharon Dalessandro, “Racial and gender differences in the returns to 2-year and 4-year degrees,” Education Economics, March 2001, pp. 281–292; Lisa Barrow and Cecilia Elena Rouse, “Do returns to schooling differ by race and ethnicity?” Working Paper 2005-02 (Federal Reserve Bank of Chicago, February 2005), pp. 34; and Lisa Barrow and Cecilia Elena Rouse, “The economic value of education by race and ethnicity,” Economic Perspectives, 2Q/2006 (Federal Reserve Bank of Chicago, 2006), pp. 14–27.
11 Barrow and Rouse, “The economic value of education,” p. 23.
12
Family background, or socioeconomic factors, may be captured through a
variety of proxies, such as income, wealth, education, etc.
13 See Current Population Survey (CPS): Statistical abstract of the United States: 2012,
“Table 229. Educational attainment by race and Hispanic origin: 1970 to
2010” and “Table 230. Educational attainment by race, Hispanic origin,
and sex: 1970 to 2010” (U.S. Census Bureau, 2012), http://www.census.gov/compendia/statab/2012/tables/12s0229.pdf; and Economic news release: college enrollment and work activity of high school graduates new release (U.S. Bureau of Labor Statistics, April 8, 2011), http://www.bls.gov/news.release/archives/hsgec_04082011.htm.
14 Thomas J. Kane, “Race, college attendance, and college completion” (U.S. Department of Education, 1994), http://eric.ed.gov/?id=ED374766.
15
Pamela R. Bennett and Yu Xie, “Revisiting racial differences in college
attendance: the role of historically Black colleges and universities,” American Sociological Review, August 2003, pp. 567–580.
16 Audrey Light and Wayne Strayer, “From Bakke to Hopwood: does race affect college attendance and completion?” Review of Economics and Statistics 84, February 2002, pp. 34–44.
17 Laura Walter Perna, “Differences in the decision to attend college among African Americans, Hispanics, and Whites,” The Journal of Higher Education special issue: “The shape of diversity” (March 2000), pp. 117–141.
18 Thomas J. Kane and Lawrence. M. Spizman, “Race, financial aid awards, and college attendance,” American Journal of Economics and Sociology, January 1994, pp. 85–96.
19 Camille Z. Charles, Vincent J. Roscigno, and Kimberly C. Torres, “Racial inequality and college attendance: the mediating role of parental investments,” Social Science Research 36, March 2007, pp. 329–352.
20 Sandra E. Black and Amir Sufi, “Who goes to college—differential enrollment by race and family background,” Working Paper No. 9310 (National Bureau of Economic Research, November 2002).
21 Philip T. Ganderton and Richard Santos, “Hispanic college attendance and completion: evidence from the high school and beyond surveys,” Economics of Education Review 14, March 1995, pp. 35–46; and Perna, “Differences in the decision to attend college.”
22 Min Zhan and Michael Sherraden, “Assets and liabilities, race/ethnicity, and children’s college education,” Children and Youth Services Review, November 2011, pp. 2168–2175.
23Grace
Kao and Jennifer S. Thompson, “Racial and ethnic stratification in
educational achievement and attainment,” Annual Review of Sociology,
August 2003, pp. 417–442.
24 Stephen V. Cameron and James J. Heckman, “The dynamics of educational attainment for Black, Hispanic, and White males,” Journal of Political Economy 109, June 2001, pp. 455–499.
25 Expenditures refer to October 2007 through December 2010.
26 A
consumer unit comprises (1) all members of a particular household who
are related by blood, marriage, adoption, or other legal arrangements;
(2) a person living alone or sharing a household with others or living
as a roomer in a private home or lodging house or in permanent living
quarters in a hotel or motel but who is financially independent; or (3)
two or more persons living together who use their income to make joint
expenditure decisions. The three major expense categories that determine financial independence are housing, food, and other living expenses. To be considered financially independent, the respondent has to provide, entirely or in part, at least two of the three major expense categories.
27 For more information on expenditures, see “Appendix A: description of the Consumer Expenditure Survey,” Consumer Expenditure Anthology, 2011, Report 1030 (U.S. Bureau of Labor Statistics, July 2011), pp. 47–48, http://www.bls.gov/cex/anthology11/csxanthol11.pdf.
28 A unique observation is also known as a “unique NEWID.” NEWID is a global identifier in CE, which identifies each observational unit.
29 For more details on balanced repeated replication, see David Swanson’s “Standard errors in the 2011 Consumer Expenditure Survey” (U.S. Bureau of Labor Statistics, 2011), http://www.bls.gov/cex/ce_se_2011.pdf.
30 The word “apart” signifies that the young adult does not live in the same household as the parent(s).
31 Tuition expenditures of type
1 households for the individual not seen in the household are captured
through a gift code, indicating whether the expenditure was for someone
outside of the household and whether it was an expenditure for college.
We do not know, however, the age of the individual for whom the
expenditure was made.
32 Data are captured through the code designating “in college full or part-time or not in college code.”
33 “Other” includes Native American (Hispanic and non-Hispanic), Pacific Islander (Hispanic and non-Hispanic), multiracial (Hispanic
and non-Hispanic), African American Hispanic, and Asian Hispanic. For
African Americans and Asians, individuals of Hispanic origin consisted
of less than 2 percent of each population.
34 Total outlays less tuition expenditures for higher education were used throughout this article. (See appendix B in this article for details.)
35 According to the “permanent-income hypothesis,” expenditures are made on the basis of levels of wealth rather than current income. Thus, total outlays indicate a consumer’s tastes and preferences better than current income does. See Milton Friedman, A Theory of the Consumption Function (Princeton, NJ: Princeton University Press, 1957), p. 6.
36 Based on Akaike information criterion (AIC), similar results were found with Bayesian information criterion (BIC), also known as the Schwarz criterion.
37 Young adults were from type 2 and type 3 households, only.
38 Results are based on logit models of college attendance on race, data controls, and family socioeconomic factors for type
3 households. Asians were consistently found to have a significantly
higher likelihood of going to college, regardless of any socioeconomic
differences. For Hispanics and African Americans, socioeconomic factors
explained some but not all of the difference in their lower likelihood
of attending college. (See appendix C, table C-1.)
39 For more information, see
“Table 2100. Race of reference person: average annual expenditures and
characteristics, Consumer Expenditure Survey, 2008” (U.S. Bureau of
Labor Statistics), http://www.bls.gov/cex/2008/Standard/race.pdf;
Table 2100. Race of reference person: average annual expenditures and
characteristics, Consumer Expenditure Survey, 2009” (U.S. Bureau of
Labor Statistics, October 2010), http://www.bls.gov/cex/2009/Standard/race.pdf; and
“Table 2100. Race of reference person: average annual expenditures and
characteristics, Consumer Expenditure Survey, 2010” (U.S. Bureau of
Labor Statistics, September 2011), http://www.bls.gov/cex/2010/Standard/race.pdf.
40 Our analyses also considered other expenses for higher education, such as room and board, textbooks. However, we did not observe any significant differences by race and ethnicity. Thus, we focused our
study of expenditures on tuition only. The results using total
expenditures, including other expenses for college, were similar.
41 Because of the limitations of the data, we could not explicitly account for differences in the amount of financial aid received across race and ethnicity,
which may affect the levels and likelihood of an out-of-pocket tuition
expenditure. A study showed that, except for Asian students, White students receive the least amount of financial aid; see Susan Borowski, “Scholarships and the White male: disadvantaged or not?” Insight into Diversity, April to May 2012, pp. 14–17, http://unival.com/PDF/InsightIntoDiversityMagazine_April-May%20Issue.pdf.
42 Data are based on quarterly outlays multiplied by 4.
43 Estimates were evaluated at annual permanent income levels between $20,000 and $120,000 for the baseline group, i.e., White, male student, parent with a bachelor’s degree, with default data controls.
44 Data were estimated for White households with a male student and an average annual income of $70,000, with default data controls.
45 Expenditures were evaluated with default data controls, as usual.
46
Zero expenditures, or “inhibitions” to observing a positive
expenditure, are essentially the first hurdle to overcome in a variation
of a double-hurdle model. However, in this article, the discussion of
zero expenditures is deferred until after a discussion of the level of
expenditures (second-hurdle in the model). (See John G. Cragg, “Some
statistical models for limited dependent variables with application to
the demand for durable goods,” Econometrica, September 1971, pp. 829–844.
47 If
a scholarship or some other kind of financial aid directly pays the
student’s tuition or reduces the tuition paid, the observed
out-of-pocket expenditure will be reduced. However, if a scholarship or
other financial aid is transferred to one’s bank account, it shows as a
source of income and the out-of-pocket expenditure will not be reduced.
48 Aid is based on each price of college. See Mark Kantrowitz, “Expected family contribution (EFC) calculator,” FinAid! The SmartStudent™ guide to financial aid, http://www.finaid.org/calculators/finaidestimate.phtml.
49
Testing for differences in the probability of having a positive tuition
expenditure between education levels indicates that parents with a
graduate degree have a significantly higher probability than those with
less than a high school diploma.
50 Scholarships
specific to minorities, as well as the effects of affirmative action,
also may contribute to a lower probability of an out-of-pocket
expenditure for non-Whites. See Borowski, “Scholarships and the White male.”
No comments:
Post a Comment